Introduction
The term dystocia is from the Greek 'dys', meaning difficult, and 'tokos', meaning birth, thus expressing a calving event with difficulty involved. The term has many subjective case definitions and tends to vary among countries. Normal calving may be defined as spontaneous calving of expected duration, which on average could be 70 minutes, ranging between 30 minutes to 4 h (Naokes et al., 2001). Sometimes, dystocia is defined as calving difficulty resulting from prolonged spontaneous calving or prolonged or severe assisted extraction (Mee, 2004). In Korean Holstein cattle, the terms calving difficulty and calving ease are used interchangeably– indicating the difficulty involved in heifers or cows while calving. The score ranges from 1 to 5 according to the level of assistance (i.e., personnel involvement or surgical removal) rendered during the calving event. The higher the score, the more severe the difficulty or less the ease at calving. Often, the phenotype scale is highly variable and even differs within and between countries.
The genetic evaluation of calving ease (CE) is critical due to the risks involved in dairy production, despite its categorical nature. To summarize the impact of CE on dairy production, it is reported that cows receiving any assistance at calving also demonstrated reduced subsequent fertility and productivity (Buckley et al., 2003). The financial impact of dystocia was also discussed by Dematawewa and Berger (1997), where it could influence production (41% of costs), fertility (34%), and cow and calf morbidity and mortality (25%). The 0ther negative impacts of CE (i.e., culling, stillbirth, respiratory, and digestive disorders) were also reported in many studies (McClintock, 2004; Mee, 2004; Lombard et al., 2007; Oltenacu et al., 1988). Many significant predictors of calving ease, such as calf birthweight, gestation length, calf gender, parity of dam, parental breeds, the climate during the last trimester, maternal nutrition, etc., were mentioned earlier in various reports (Johanson & Berger, 2003; Philipsson, 1976; Meijering, 1984; McClintock, 2004; Kertz et al., 1997; Berger et al., 1992). Previously, Alam et al. (2017) also estimated the genetic parameters of CE in Korean Holstein heifers. However, they did not elaborate on the individual predictors responsible for CE.
In South Korea, the evaluation of CE is particularly important for an0ther reason. As a member country in interbull genetic evaluation, integrating CE in the Korean national evaluation model alongside major production traits would also enable Korean breeders to compare Korean bulls' genetic ability with 0ther international Holstein bulls. However, no national evaluation model accounts for the CE trait in Korean Holsteins. For this reason, investigating and selecting primary model terms or systematic factors responsible for CE is a prerequisite and will be a significant first step in further evaluations. It would allow the formulation of future genetic evaluation models for CE. Therefore, this study aimed to investigate the distribution of CE, identify the importance of various genetic and non-genetic factors, and propose a list of factors for future CE trait evaluation in Korean Holstein cattle.
Materials and Methods
1. Animals, calving ease scores, linear transformation of scores, and data screening
A total of 83,943, 69,120, 31,500, 29,003, and 9,361 CE observations in the first five parity Korean Holstein cows were analyzed. The CE data, collected between 2000 and 2021, was provided by the Dairy Cattle Improvement Center (DCIC) in the Republic of Korea. As mentioned earlier, the CE phenotype is comprised of five levels such as CE of 1 (non-assisted calving), 2 (slightly assisted calving: 1 person), 3 (moderately assisted calving: 2 to 3 persons), 4 (difficult calving: >3 persons), 5 (extremely difficult calving or surgical removal involved). We also performed a linear transformation of the discrete CEs via the snell scoring method (Snell, 1964; Alam et al., 2017) for each parity phenotype (Table 1). Some details on the snell score transformation are given by Alam et al. (2017). To summarize, this conversion would account for an underlying continuous distribution of CE phenotype and help attain a better normal distribution instead of a skewed distribution of CE. The snell procedure assumes a latent continuous scale between the interval of ordered categories, and each transformed score represents the middle of the interval. We, however, forced the score range of calving ease to scale between 0 to 100%, such that a score of 0% would express the least calving difficulty (CE of 1) and 100% would denote the highest calving difficulty (CE of 5), but in a continuous uneven scale between levels. The final datasets are prepared based on the following restrictions. Only single-born calving events were allowed to stay in the dataset. The final datasets consist of calving records between 260 and 296 days of gestation lengths. The calving age was also constrained between 20 & 36 mo, 30 & 54 mo, 42 & 66 mo, 54 & 78 mo, and 66 & 90 mo for 1st, 2nd, 3rd, 4th, and 5th parities, respectively.
Table 1. Estimates of linear transformed calving ease scores in actual snell and percent snell scales ![]() |
2. Factors of interest
We considered several genetic and non-genetic predictors (factors) of calving ease for fitting into the statistical models. These predictors were calf sex, calf size at birth, calving herd (farm), calving year, calving season, gestation length of the dam, and calving age. The fixed effect of calf sex (SEX) was fitted, assuming a potential influence of progeny's sex on the calving event. For calf size (SIZE) as a fixed effect, the effect included three categories- small, medium, and large birth sizes based on the farmer observations during the calving event. Generally, calf birth weights are known to be more closely related to the calf's physical dimension. However, as the present study lacks calf birthweight information, we assumed the calf size as a reasonable proxy to the birthweights as a predictor. The gestation length of the dam, between 260 to 296 days, was converted into three categories, i.e., short (<275 days), normal (275-281 days), and long (>281 days). The fixed effect of dam calving age (DAGE), grouped by month, was included to identify any possible influence of a cow's maturity level on her subsequent calving event. As for non-genetic predictors of CE, the effects of farms/herd (HERD), calving year (YR), and calving season (SEA) were fitted as fixed effects. Given the large number of levels for the calving herd-year-season effect (HYS), typically included in many dairy genetic evaluation models, it was computationally challenging to fit any statistical model in this study. We, however, controlled for HYS and reduced the herd numbers across parities. The restriction includes a minimum of two records (parity 4 and 5) and three records (parity 1 to 3) per HYS group. This restriction allowed the statistical computation to be feasible with models including the herd effect. The slight ease of restriction in later parities was due to lesser records in those datasets.
3. Statistical Analysis
1) Relationships among predictor variables
Before statistical significance testing, the variance inflation factor (VIF) analyses were performed on each dataset via fitting a GLM model CE as a response with all predictors except herd effect due to its large levels. The glm function in R (http://www.r-project.org/) and the vif function in the package 'car' in R (Fox & Weisberg, 2019) was used for the VIF analysis. The VIF analysis is used to detect any severe multicollinearity between predictor variables. Any predictors exceeding a VIF value of 4 were considered for removal for further data analyses as a general rule of thumb. Our VIF analysis showed that most predictors had an acceptable range of relationships in each parity dataset, therefore, were not excluded from further analysis (Table 2).
2) Descriptive statistics
Detailed descriptive analysis on a single parity dataset was performed for calf sex, calf size, calving season, calving age, and gestation lengths. Descriptive statistics and figures were generated using the package 'dplyr' (Wickham et al., 2022) and 'ggplot2' (Wickham, 2016) in R. In some graphs, the CE3, CE4, and CE5 categories were combined into one as CE3+ due to low frequency of phenotypes in respective categories.
3) Generalized linear regression using the actual CE scores
The distribution of CE scores is generally right-skewed. Despite the non-normal distribution, our first GLM model fitted the CE trait with predictors assuming a linear relationship between all predictors and the response variable CE. The significance of predictors for all parity datasets was estimated separately using the same model. The model included SEX, SIZE, GLEN, HERD, YR, SEA, and DAGE fixed effects. No random effects were fitted in this model. The glm function distributed under the R software package used GLM analysis. The GLM model of analysis was as follows:
Yijklmno = µ + SEXi + SIZEj + HERDk + YRl + SEAm + GLENn + DAGEo + eijklmno
where yijklmno is the CE of any parity, µ is the intercept, SEX is the effect of ith level of calf-sex, SIZE is the effect of jth level of calf-size, HERD is the effect of kth level of calving herd, YR is the effect of 1th level of calving year, SEA is the mth effect of calving season, GLEN is the effect of nth level of gestation length group, DAGE is the effect of 0th level of monthly calving age groups, and eijklmno is the residual term.
4) Generalized linear regression using the linear transformed (snell) scores
However, this analysis replaced the evenly-spaced categorical CE levels with unevenly-spaced linear transformed CE scores. The GLM model fit for linear-transformed CE trait also included the similar predictor variables required for the original score-based analysis. The parameters and significance statistics of this GLM fit were compared with the previous GLM estimates.
5) Stepwise generalized linear regression analysis
We performed stepwise regression analyses for all datasets using the complete model and searched for the best models with meaningful predictors. The step function in R was used for stepwise GLM regressions. The model with the lowest AIC values was considered the best for individual parity analysis.
6) Relative importance of predictor variables
We assessed the relative importance of detected significant variables in parity-wise datasets by using a type='lmg' option in the 'relaimpo' package in R (Grömping, 2006), which is based on the 'averaging over orderings of regressors' procedure. The 'ggplot2' package in R was used for producing the related barplot using original CE datasets.
Results
1. Distribution of calving difficulty by predictors
The distribution of CE by calf sex across parity is presented in Table 3. Within parity datasets, the ratio of males and females number deemed somewhat close to each 0ther. Between parity datasets, the male birth (%) was deemed slightly higher in later parities, whereas, relatively lower for female calves. Given the higher percentage of males in the later parties of dam, it could also reflect greater calving difficulties in those lactations (Figure 1). The parity-wise distribution of CE by calf-size groups (Table 4) showed that the proportion of medium-sized (normal) calves born was much greater (>92%) than the total of small- or large-sized calves (<8%). Over the parity, the percentage of small-sized calves increased by a few percentage points, whereas large-calf ratios remained somewhat stable. Therefore, it is expected that the more male calves in later parities could also be related to greater difficulty calving. For example, larger-sized calves across parities were more subjected to CE2 or higher than large female calves in that group (Figure 2). In later parities, higher male proportions, irrespective of their sizes, were related to CE3 and greater difficulties. The mean calving age, shown in Table 5, indicates that the second and higher parity cows were more variable (SD of ±132.0 to 147.7 days) in calving ages than heifers (SD of ± 91.8 days). Cows' calving ages also vary more when the dam ages. The average calving age in heifers was 26.3 months, whereas 80.9 months in the fifth parity. Figures 3 and 4 show the distribution of CE according to the calving age of dams. Overall, CE3+ increased over the dam's parity. However, there was no distinct pattern of CE3+ within parity due to calving ages, where the first three parities showed opposite trends than the following two parities. Probably this is because of smaller datasets in parity 4 and 5.
Table 3. Distribution of male and female calves in Korean Holstein according to parity1 ![]() |
1Value without and within parentheses indicate the total number of observations and % observations |
Table 4. Distribution of calf size at birth in Korean Holstein according to parity1 ![]() |
1 Value without and within parentheses indicate the total number of observations and % observations |
The average gestation length variation in Korean Holstein was stable across parities (SD of ± 5.2 to 5.3 days) in Table 6. The average GLEN increased almost consistently with the dams' age. The lowest average GLEN in dams was identified in the first parity (276.8 days), whereas the highest average GLEN was found in parity-5 dams (280.0 days). More unexpected calving scenarios, i.e., CE3+, strongly followed longer gestation lengths than normal calvings (Figures 5 & 6). Our study on GLEN with 0ther factors also revealed some patterns. The SEX by GLEN investigation shows that male-calf births were more associated with longer GLEN than days required for female-calf birth (Figure 7). For SIZE by GLEN analysis, a shorter or longer gestation length was found to be related to non-normal-sized calves (Figure 8). It was also evident that cows' calving ages varied the least with normal gestation periods, irrespective of parities (Figure 9). Any shorter or longer gestation length is associated with higher calving age diversity in dams. The calving year by CE trend showed that calving ease increased over time (Figure 10). Table 7 shows the distribution of calf births according to season. The seasonal calving records in the first parity were similar (24 to 26%). It was parity two and onwards, where autumn calving records increased noticeably. However, there were no distinct patterns regarding the percent CE3+ incidence according to season (Figure 11).
Table 7. Distribution of calving season in Korean Holstein according to parity1 ![]() |
1Value without and within parentheses indicate the total number of observations and % observations |
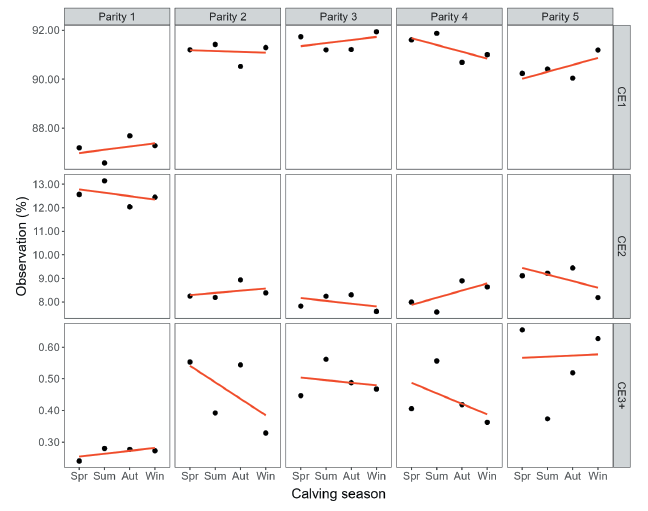
Fig. 11. Percentage observations of calving ease by calving season and parity (CE1, normal calving; CE2, slight assistance; CE3+, moderate, difficult, and extreme difficulty calving combined; Spr- spring [March-May]; Sumsummer [June-August]; Aut- autumn [September-November]; Win: winter [December-February]).
2. Statistical significance of predictors
Tables 8 and 9 show the list of significant predictors from the GLM regression (complete model) based on actual and linear transformed CEs. A1though there were some differences in regression estimates and p-values for predictor variables in b0th actual and transformed CE analyses, the list of significance predictors remained similar for individual parity datasets. The effect of calf sex was significant (p<0.05) in most cases except for the third parity. The snell-transformed regression estimate indicates a 10% increase in calving difficulty if the calf born was a male. However, the calf size effect was highly significant in all parities for any CE datatype. The GLEN effect was more important in heifers than older cows. However, the DAGE effect was non-significant for CE phenotype in all the parity. The calving herd effect was always significant, whereas the calving year was also significant in most parity analyses. An exception was the calving season effect which demonstrated non-significant influences on CE.
Table 8. Significance of factors using GLM model based on actual CE datasets1 ![]() |
1NS- non significant at P <0.05 |
Table 9. Significance of factors using GLM model based on linear transformed CE datasets1 ![]() |
1NS- non significant at P <0.05 |
3. Stepwise regression analysis and relative importance of effects
The stepwise regression summary is presented in Tables 10 to 12 based on actual and linear transformed scores. From the complete model analyses, the list of significant factors for parity-wise datasets varied to some extent (Table 10). The effect of SIZE, HERD, and YR remained significant across all dataset analyses. However, the SEX effect was removed from the third parity only. Similarly, the GLEN effect was dropped from models for the third and fourth parity analyses. SEA was only significant in the second parity analysis. The DAGE effect is removed from all parity final models via stepwise method. The retained lists of significant effects were identical parity-wise. A1though there were noticeable differences for the adjusted R-squared values across parities (0.30 to 0.51) between CE datatypes analyses, the model fit estimates were relatively close.
The relative importance of significant factors for parity 1 to 5 datasets using actual scores is shown in Figure 12. The effect of HERD appears to be the most crucial effect of all. The second substantial effect is SIZE, followed by the YR effect. However, SEX and GLEN contributed relatively less in the final models.
Table 10. Factors selected by stepwise GLM model based on actual or linear-transformed CE datasets1 ![]() |
1Factor retained (+) or removed (–) |
Discussion
In this study, we assumed calf sex (gender) to have influences on CE. In this regard, our research showed a general relationship between male calves and higher CE scores from second parity onwards, i.e., CE of 2 and greater (Figures 1 and 2). These figures depict the greater risks of calving difficulty in males in later parities. Mee (2008) stated some reasons for CE trends in males. One reason is the morphology, where the calf is a male gender itself can contribute to an increased risk of dystocia for itself. Berger et al. (1992) showed higher dystocia rates in males irrespective of birth weight ranges. An0ther reason is that male calves are generally higher in body weight than female calves. The study by Johanson and Berger, 2003 agreed with a similar cause and attributed the higher male body weights to 25% higher odds of dystocia in their male calves. In our study, the higher proportions of larger male calves in CE2 and CE3+ groups of all parities also support the male sex and its CE association (Figure 2). More investigation into the Korean Holstein dataset reveals that larger and average-sized male calves can experience severe difficulty at birth if their dams are old. The effect of sex was also significant in the present study. Despite the lower relative importance of calf sex on CE (Figure 12), their overall significance across parities indicates their inclusion potential in the genetic evaluation models of Korean Holstein.
Previously many studies suggested the importance of birthweight for dystocia (Mee, 2008; Johanson & Berger, 2003; Menissier & Foulley, 1979) and further stated its relationships with gestation length, parity, fetal gender, parent's breeds, etc. (Mee, 2008). Due to a lack of birthweight information, we assumed the calf-size group to be closely related to CE. We expected that a larger calf size would closely represent their higher birthweights, resulting in greater calving difficulties. While strongly supporting our assumptions, the calf-size effect significantly influenced CE. The larger calf sizes across parities were also considerably associated with more difficult calvings. Therefore, we could draw some indirect agreements with earlier reports which studied the birthweight of calves instead of calf sizes. The overall significance of the calf-size effect and its reasonably high relative importance in the model also suggests its inclusion potential for Korean Holstein evaluations. While many studies included birthweight in their genetic models for dystocia, it is not beyond any discrepancy, especially if it is an estimate (Phocas & Laloe, 2004; Tomka, 2018). Note that birthweight and calf size are not easy to measure observations, especially during calf birth. Therefore, sometimes these observations could be estimated instead of measured, as illustrated by Phocas and Laloe, 2004 for birth weight. The calf size in this study is an estimate primarily based on farmers' experiences– because no definition of calf size is available currently. As a result, there is a possibility for bias in calf-size categories among herds. For example, a farmer may report consistent values due to inadequate distinction between categories. It, thus, poses a risk of losing potential within herd variations (Tomka, 2018). Also, a particular calf-size category seen by one farmer may be different from the same value by an0ther farmer. While the between-herd bias can be minimized by combining herd and calf size into one factor, the within-herd bias is difficult to control until calf size is defined strictly. Therefore, we suggest further investigation into within-herd distribution for calf size and placing restrictions on records if a pattern exists.
The above-discussed effects (for example, sex and size) are closely related to the gestation length of the dam. Our study found evidence of female calves requiring a consistently lower gestation period than males (Figure 7). Similarly, the larger calf-size group is associated with longer gestation lengths (Figure 8). Further evidence of a higher incidence of difficulty calving (CE3+) at longer gestation periods across parities is found in the present study (Figure 5). Our findings were deemed in some agreement with Philipson (1976), who only reported an association of b0th short ( <265 days) and long ( >285 days) gestation lengths with increased dystocia in first parity dams (Philipsson, 1976). According to their report, dystocia at shorter gestation periods is due to fetal mortality, twins, and premature calving, and at longer periods due to fetal oversize. Literature suggests that fetal growth rate averages could be as much as 0.3–0.4 kg/day during the last month of gestation (Meijering, 1984). It indicates that a longer gestation period assisting fetal oversize can eventually increase difficulty at calving. In our study, b0th short and long gestation lengths proportions have increased over parity and indicated a higher incidence of calving difficulty. On the 0ther hand, the effect of GLEN being significant across multiple parities alongside its relative importance in statistical models suggest that this effect is a candidate for inclusion in the final genetic evaluation models for CE improvement. Olson et al. (2009) added that the parity of calving could affect the dystocia occurrence through shorter gestation and lower calf weights in heifers.
Earlier literature proved that the incidence of dystocia differs according to the parity of the calving (Berglund, 2008; Atashi et al., 2012; De Amicis et al., 2018). Generally, the existing literature emphasizes the difference between the first and further calvings. According to Juozaitiene et al. (2017), most of their cows experiencing difficult calving in early lactation also displayed difficult calving in consecutive calvings. In this study, we also find that proportion of difficult calvings increased in later parities (Figure 3). This finding is supported by Mõtus et al. (2017), who also observed higher dystocia in the third and later parities than in the second parity. The calving difficulty could differ based on age and cow parity. For heifers, the smaller pelvic size is the reason which leads to calving difficulties (Fuerst & Egger-Danner, 2003). For older cows, in contrast, it is the malpresentation of the calf, weak labor, and insufficient dilatation of the cervix which lead to the calving difficulty (Meijering, 1984). Calving age in this study also showed the between-parity trends for calving ease according to age variations. Cows tend to calve with more difficulty (CE3 and above) in the first three parities. The reason for the lower percentage in parity 4 and 5 could be a relatively low amount of records. However, despite some phenotypic trends for calving age and CE, we could not find support for significant differences for the calving age group within parity. The reason could be partly due to the absorption of some variances by the gestation length predictor. It is because calving age also includes gestation length by definition. Therefore, instead of fitting a fixed calving age group effect, a control for actual calving age (in days) as a fixed covariate might improve the model parameters.
We also fitted the effects of calving herd, year, and season as independent effects. Our study showed the significance of HERD effect on CE. HERD also had the most crucial effect on Korean Holsteins. Generally, a herd contributes to CE via management differences. Holmøy et al., 2017 mention that herd management is a critical factor in influencing heifer dystocia incidences and, therefore, adequate service weight of the heifers at the first mating. Our study also showed that the significant influence of the calving year. Overall, this study had an increased calving ease performance (higher CE1) over the years, and this is probably due to improved managements. Alam et al. (2017), who studied only heifers from the same Holstein population, also reported a similar pattern a few years ago. The present reports also agree with Hansen et al. (2004), where a decline in greater calving difficulties was reported. The calving season effect, in contrast, was found non-significant in this study. It disagrees with many 0ther reports where a similar factor was identified as significant (Meyer et al., 2000; Fuerst & Egger-Danner, 2003; Matilainen et al., 2009). A1though there was some support for winter and spring (in parity 2 and 5) for higher rates of CE3+ with 0thers (Uematsu et al., 2013; Mekonnen & Moges, 2016), overall, there were no definite trends. According to Tomka (2018), cows experiencing the last part of gestation in the winter and having an improved feeding regime could promote greater fetal growth leading to calving difficulties. While a similar feeding regime pattern may be somewhat likely in Korean cattle during winter but may not have enough influence on causing noticeable calving differences. However, it is to note that we implemented restrictions on herd records to overcome the computational limitations with a large number of herd levels, which also excluded difficulty calving records noticeably. An analysis using a much larger dataset may produce different outcomes as well. Therefore, we suggest using the combined contemporary group effect of calving herd, year, and season for effective separation of the management and environmental contributions to CE in future genetic analyses. In this study, the list of significant factors differed slightly across parities. The differences are probably due to graters variations in data sizes across parities. However, our outcomes remained identical irrespective of the datatypes used, such as actual CE or linear transformed CE scores. Some model parameters, such as adjusted R-squared estimates from linear transformed CE analysis being slightly higher, may indicate its appropriateness over the actual score. Despite the method of choice for evaluation– which could be an0ther research topic in the future– the set of predictor variables identified here could be included in any models for CE evaluations. We suggest that some predictors such as calving herd, year, and seasons are used as a combined 'herd-year-season' effect in genetic models, even though season showed statistical non-significance at p<0.05. We also emphasize the further investigation of calf size with respect to herd due to its nature, as discussed earlier, to reduce any measurement bias. The fixed effect of DAGE could be removed from a statistical model if gestation length is already included. However, the calving age as a fixed-covariate could be examined further and included as a control variable when appropriate. We believe that the outcomes of our study could help design future genetic evaluation models in Korea.
CONCLUSIONS
The present study aimed to understand the distribution patterns of calving ease (or difficulty) across parity of Korean Holstein and identify factors influencing the trait. A1though CE was observed irrespective of levels of predictors or parities, there is evidence of specific patterns in occurrences. Male calves were primarily associated with longer gestation lengths and greater calf sizes and assisted more towards difficult calving. Oppositely, female calves showed calving difficulty due to shorter gestation length and being smaller in size. Overall, females calves with CE3+ were relatively less abundant than males. Gestation lengths of dams also increased as parity advanced. Thus, longer gestation lengths promoted higher calving difficulties in Korean Holstein, irrespective of calf sex or size. The calving age of the dam demonstrated no significance, whereas calf size and sex were statistically significant for CE evaluation. The statistical importance of the herd effect was the most among all predictors. Despite some differences in statistical parameter estimates, the statistical significance for predictor variables using actual and linear transformed phenotypes was nearly identical. Our present outcomes will be essential resources for future genetic evaluation models regarding calving difficulty in Korean Holstein cattle.
ACKNOWLEDGMENTS
This work was carried out with the support of "Improvement of national livestock breeding system and advancement of genetic evaluation technology (Project No. PJ016703042022)" from the Rural Development Administration, Korea. This study was supported by the RDA Research Associate Fellowship Program of National Institute of Animal Science, Rural Development Administration, Korea.